Still-life artists know that to make an image of an object look like the real thing, they must account for the way light reflects off it. The appearance of these glimmers—their color, position and brightness—is influenced by the item’s surroundings. And this effect means an object can, in turn, reveal key aspects of its environment. Researchers have now found that by filming a brief video clip of a shiny item, they can use the light flashing off it to construct a rough picture of the room around it. The results are surprisingly accurate, whether the reflections come from a bowl, a cylinder or a crinkly bag of potato chips.
The mathematical model used to reconstruct environments can also approximate what a known object will look like—how light will reflect off it—when it is placed in new surroundings or is seen from a new angle. These two applications are linked. “The challenge of our research area is that everything so entangled,” says Jeong Joon Park, a Ph.D. student at the University of Washington’s Graphics and Imaging Laboratory (GRAIL). “You need to solve for lighting to get the good appearance. You need to have a good appearance model to get the good lighting. The answer might be to solve them all together—like we did.”
Park’s team posted a preprint of its study on the server arXiv.org earlier this year. And the paper was also accepted for presentation at the next annual IEEE Conference on Computer Vision and Pattern Recognition, which will be held remotely in June.
Park says the new work could help designers build more realistic augmented- and virtual-reality landscapes. For example, on Zoom video calls, many people apply artificial backgrounds with lighting that does not match the illumination of the actual user. Environment-reconstructing technology could modify a backdrop to make a real face fit better with virtual surroundings.
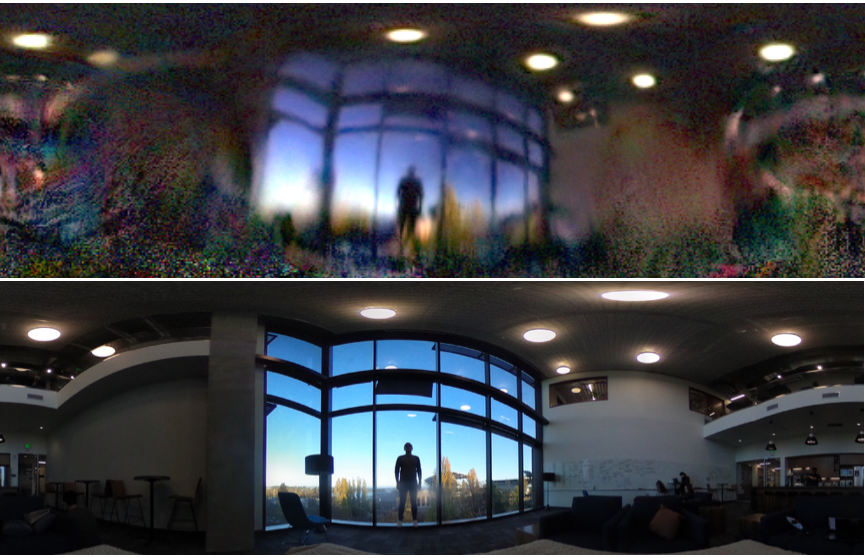
In another example, an augmented-reality app might struggle to portray how a piece of furniture would look in a user’s living room: it might appear more like a cartoon cutout than a solid fixture. Park says his team’s model could make it seem as if the item is actually in the room. “You must have some estimate of your environment to currently light this virtual object,” he says. “And the more accurate [the lighting] gets, the better the virtual object will be.”
This technology also has applications for virtual reality. In a VR landscape, users might walk around an artificial scene while wearing a headset or “pick up” a digital artifact and turn it over in their hands. When they do so, the way that item looks should change—as it would in the real world—because of ambient light conditions. Park says his team’s system can calculate the character of that light to “give you a very realistic estimate of the appearance of any viewpoint of the scene.”
This process is called view reconstruction, or novel view synthesis. Park’s team is trying “to come up with a way to synthesize or to generate a view of an object that you haven’t seen before,” says Jean-François Lalonde, an associate professor of electrical and computer engineering at Laval University in Quebec, who was not involved in the new study. “You observe a particular object from different orientations. And then you want to know what that object would have looked like if you were to see it from a different orientation that you didn’t see before.” Previous research tackled the problem with two separate techniques, Lalonde explains. The earliest attempts used an object’s geometry, along with physical laws, to calculate how light and color would change as the view shifted. Then, “for the past two or three years or so, we’ve seen another set of approaches that tried to come up with a different way of modeling the world,” he says. The more recent methods used deep-learning algorithms to memorize what an object looked like and project how its appearance would change under a different view.
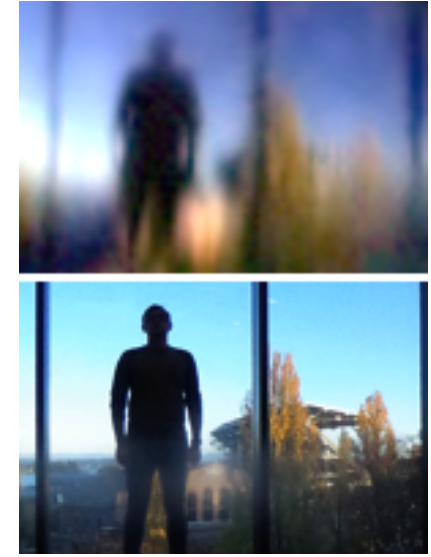
The new study relies on a combination of both types of techniques. Doing so lets the researchers “exploit the strength of the physical reasoning, along with the power of statistical reasoning that comes with deep learning,” Lalonde says. “They’re embedding even more physical reasoning in the [deep] learning process, and that allows them to get higher-quality results.”
Park and his colleagues put their novel view synthesis method to the test by using it to reconstruct images of the surrounding environment. They employed a video camera to film a variety of items—the aforementioned bag of chips, as well as soda cans, ceramic bowls and even a cat statue—then reconstructed the environment that produced those reflections with their model. The results were remarkably true to life. And more predictably, mirrorlike objects produced the most accurate images. “At first, we were pretty surprised because some of the environments we recovered have details that we cannot really recognize by looking at the bag of chips with our naked eye,” Park says.
He acknowledges that this technology has an obvious downside: the potential to turn an innocuous photograph into a violation of privacy. If researchers could perfectly reconstruct an environment based on reflections, any image containing a shiny object might inadvertently reveal much more than the photographer intended. Park suggests that as the technology improves, its developers should bear this problem in mind and proactively work to prevent privacy violations.
He also notes, however, that the images are relatively unclear for now. “I hope that future works will build on our work to improve the quality of both the reconstruction of the environment and the scene,” Park says. “My long-term goal is to reconstruct the real world. And that includes not only good visualization but also being able to interact with virtual environments.”
"can" - Google News
May 04, 2020 at 06:32PM
https://ift.tt/35rPEQK
A Shiny Snack Bag's Reflections Can Reconstruct the Room around It - Scientific American
"can" - Google News
https://ift.tt/2NE2i6G
https://ift.tt/3d3vX4n
Bagikan Berita Ini
0 Response to "A Shiny Snack Bag's Reflections Can Reconstruct the Room around It - Scientific American"
Post a Comment